LLMs vs. MLLMs: The Future of Language Modeling in AI
Explore how LLMs and MLLMs are reshaping AI by advancing language and multimodal capabilities to revolutionize tech across industries.
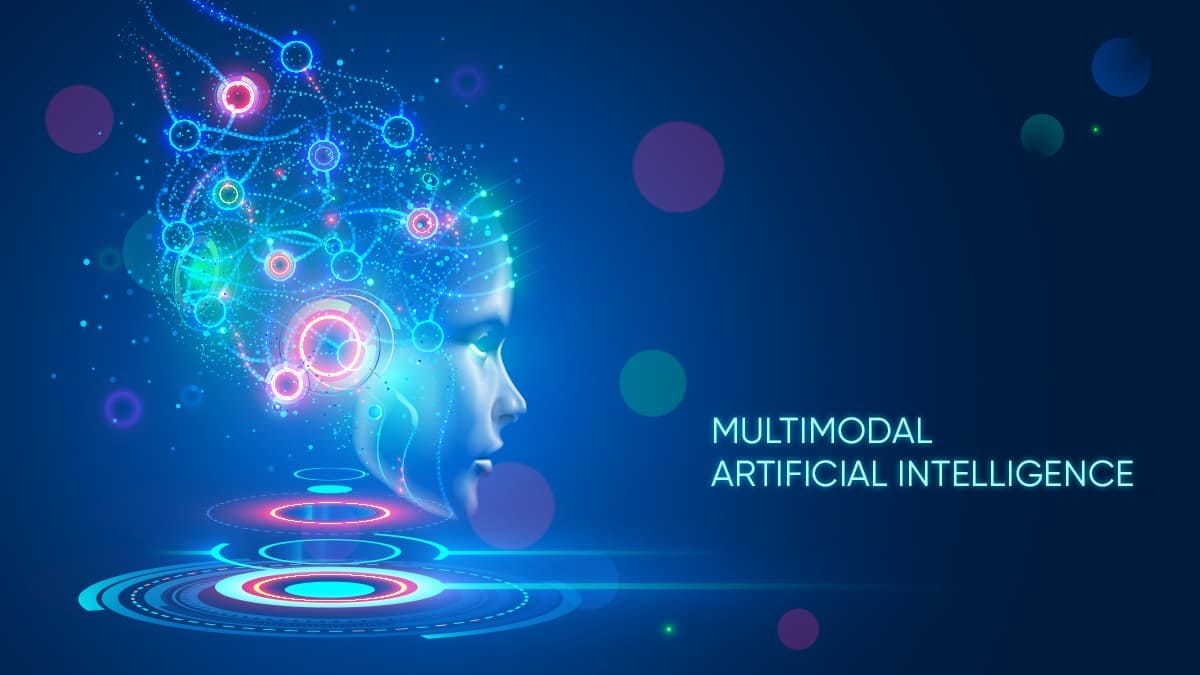
Artificial Intelligence is the newly turning face of interaction with technology, structuring industries in ways unimaginable. Two large model types, the Large Language Models or LLMs, and another called Multimodal Large Language Models or MLLMs, are at the forefront of this revolution in AI. Despite their predominantly similar-sounding names, both these models undertake very different purposes in the AI environment. We shall outline what LLMs and MLLMs are, how they differ from each other, and why they are assumed to be the necessary driving factors for the development of the next generation of AI applications.
LLMs deal with language. AI models that train with or generate data in other modes—such as audio, images, or specialized data like DNA sequences—are known as multimodal large language models, or MLLMs.
The Basics: Large Language Models (LLMs)
LLMs are a class of foundational AI models that only take input in the form of language. Built from billions of text data points, the training of LLMs to read, understand, generate, and translate text involves complex probabilistic algorithms that can grasp even the subtleties of language. It captures patterns using sentence structure, grammar, and even cultural context. This enables them to create human-sounding text. Applications include conversational bots, voice assistants, content creation tools, and machine translation systems.
The result of such training on vast amounts of text data has equipped the LLMs with the capability to produce highly coherent and contextually relevant text. However, the LLMs are confined to processing and generating text-based results only, as they cannot directly interact with any other form of data, such as images or audio. This is where the next evolution is known as MLLMs.
Enter MLLMs: Expanding Beyond Text
While LLMs vary in their cores, Multimodal Large Language Models add the power of processing more than one type of data to the capabilities of LLMs. MLLMs are multimodal because of training on data sets, including text, pictures, audio, and even code, that would adapt it for applications where text analysis would not be sufficient. For instance, an MLLM might take a text description as input and generate an image, or it could analyze a screenshot and then write HTML to match it. Others might read input from audio and provide a relevant text response to what was said.
This multimodal approach gives MLLMs the advantage in the performance fields that require integrating and interpreting complex data, such as autonomous driving, medical image recognition, and high-degree visual recognition systems. The design of MLLMs goes beyond just "seeing" or "hearing" to carry out applications that synthesize jobs from many forms of input for more accurate output.
Behind the Scenes: Training LLMs and MLLMs
Both LLMs and MLLMs require enormous amounts of data and computing to attain these capabilities. Such training methodologies require feeding these models with large volumes of information from diverse sources, a cumbersome task that demands pre-processing, refinement, and continuous iterations to improve their accuracy. While both deep learning model types require quality data, MLLMs face added challenges as different data types are integrated, typically requiring complex alignment and synchronized training.
Advances in hardware and storage solutions have also made LLMs and MLLMs feasible. For instance, high-performance storage solutions such as Pure Storage with NVIDIA's GPUs tuned LLMs and MLLMs for faster training. They gave them the capability to handle increasingly complex data pipelines. Pipelines like RAG enhance model accuracy and relevance further by allowing LLMS to refer to external sources in real-time and even improve the model response through those references.
Real-World Applications: Where You’ll Find LLMs and MLLMs in Action
They already power digital assistants, chatbots, and content creation tools for users to find information, customer service, and even creative writing. High-profile examples of LLMs include OpenAI's GPT models powering ChatGPT and Meta's Llama series.
Because MLLMs can process multiple data types, greater possibilities open up. For instance, Gemini MLLM from Google DeepMind integrates data from text, code, audio, images, and videos into one, making it perfect for website design, from screenshot inputs to summarization of video input in a textual format. By handling data from various domains, MLLMs can support other innovations such as autonomous vehicles, medical imaging, and visual arts.
Optimizing LLMs for Enterprise Use: RAG and Beyond
Such RAG pipelines with LLM make much sense and add value in an enterprise setting where specificity and relevance count. Integrating domain-specific, external data into the AI model educates the AI for responses to become more specific and relevant to actual business scenarios. Merging those with NVIDIA's Enterprise-class, high-performance GPU and networking, combined with Pure Storage's FlashBlade technology, provides an enterprise with the best performance at scale while considering the accuracy of data for its LLM solutions.
LLMs vs. MLLMs: Which Model is Right for You?
Choosing either LLMs or MLLMs depends on how simple or complex your use case is. LLMs will suffice for complicated text analyses, translation, and generation. Suppose the project requires integrating numerous data types, such as audio, images, and video. In that case, MLLMs possess the much-required flexibility and deepness to generate or process information from diverse inputs.
With the development of AI technology, the gap between LLM and MLLM will always be minimal. Researchers and developers continuously try various means of integrating multimodal capacity into typical LLM and vice versa to develop more robust AI solutions.
Conclusion
Improvements within large language models and MLMs underline AI's capability to solve more complex tasks. Due to their deep comprehension of language, large language models have replaced the way we used to interact with technology by making our applications more conversational, accurate, and responsive than ever. Meanwhile, it's worth noting that MLLMs extend these features by incorporating various data types and, thus, combinations of text, images, audio, and other forms in one unified AI experience to enable applications ranging from self-driving cars to medical imaging.
Consequently, understanding the conceptual distinctions between an LLM and a many-task model becomes paramount for leveraging AI for competitive advantage. As much as LLMs are outstanding in their deeply specialized narrow Art, MLLM opens the door to combined interaction with other modalities to unleash innovative technologies across various industries, verticals, and applications.
AI's future is multimodal, promising even more accessible and more intuitive technologies that can understand and respond to the full spectrum of human communication and data. Properly selecting models for specific needs will enable enterprises to exploit such a development to provide user-friendly, efficient, and powerful AI-driven solutions.